Chinmaya Kausik
Mathematics Ph.D. Student, University of Michigan.
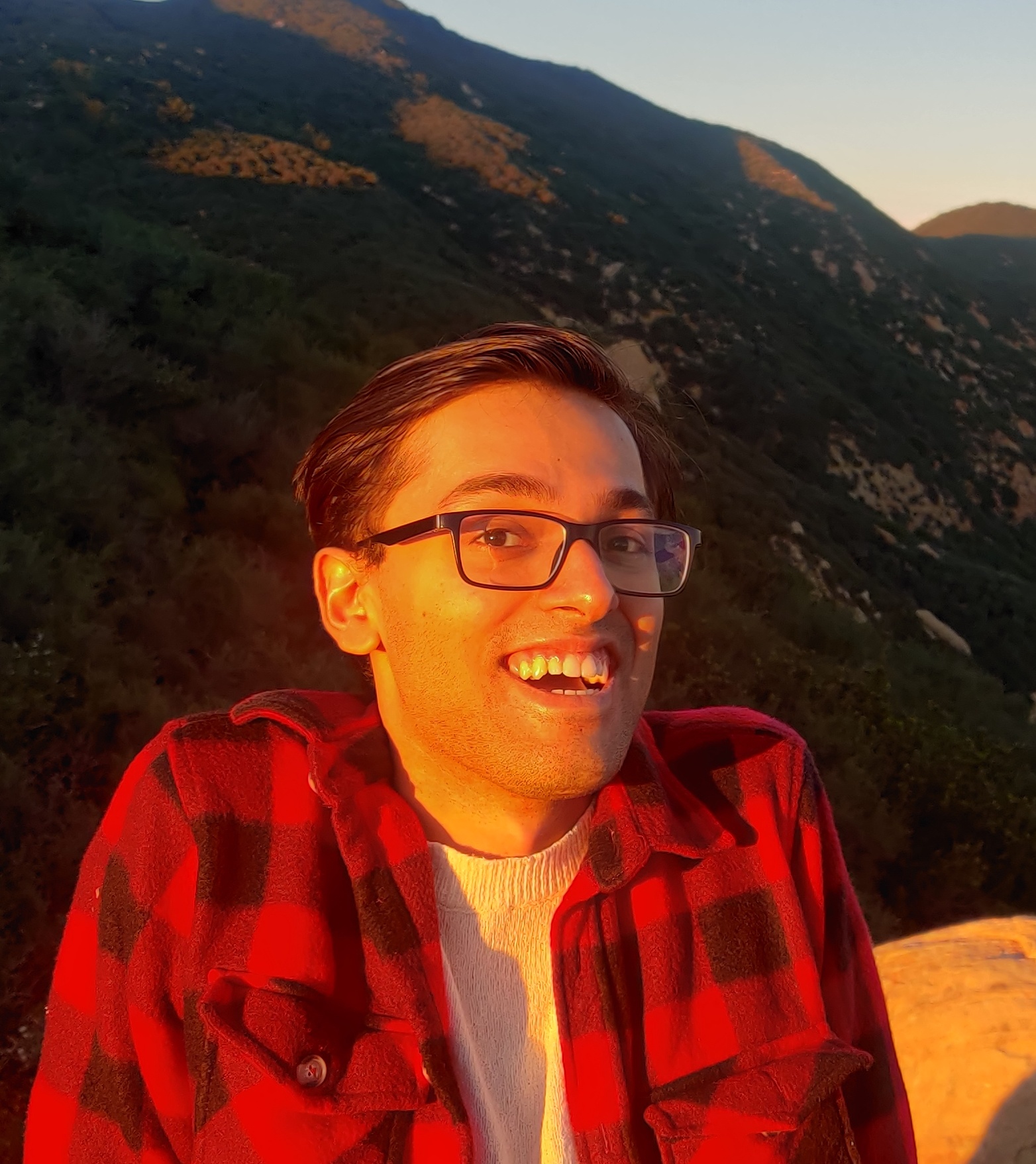
Hi there! I’m Chinmaya Kausik, a 4th year mathematics Ph.D. candidate at UMich working on machine learning, statistics, optimization and sequential decision-making. I am being co-advised by Prof. Ambuj Tewari and Prof. Martin Strauss.
I design and implement principled algorithms and agents, and provide theoretical and empirical guarantees on their performance. My work spans reinforcement learning, bandits, RLHF, and designing as well as post-training LLM agents. I am also working on personal projects involving other aspects of sequence models like LLMs, transformers, state space models.
You can find my resume at this link. Check out my papers, projects, and personal interests!
What do I care about, broadly?
- Tackling tangible, real-world questions with a principled mathematical approach. These days, my PhD research focuses on sequential decision making under various settings - offline-to-online transfer, partial observability/latent information and non-standard feedback and reward models. I also have side projects and internship research in deep learning, LLM agents, transformers, Bayesian inference, etc. On the other hand, a lot of my undergraduate background was in geometry, topology and dynamics, with work in computer-assisted topology and geometry.
- Increasing accessibility to and in higher mathematics and creating communities where ideas cross pollinate and people pull each other up. I have started the Stats, Physics, Astronomy, Math (SPAM) graduate student social initiative at the University of Michigan. I also co-founded and co-organize Monsoon Math Camp. I have also been involved in building and expanding other mathematical communities, like platforms for the PolyMath REU, DRP programs and the undergraduate math organization at IISc, etc.
What am I doing these days?
- Working on my internship at Netflix, where I am focusing on post-training LLM agents to help them reason about very long contexts! This would apply to helping SWE agents work with large codebases, helping generative recommenders handle massive catalogues, etc.
- Writing a paper based on my internship at Microsoft in the advertiser optimization team under Ajith Moparthi! I designed and implemented a fast algorithm for updating models used for advertiser bidding.
- Collaborating with Yonathan Efroni (Meta), Aadirupa Saha (Apple), Nadav Merlis (ENSEA) on algorithms for bandit and reinforcement learning algorithms with feedback at varying costs and accuracies, also called multi-fidelity feedback.
- Thinking about principled approaches to data collection and learning for RLHF under real-world considerations.
- Formulating problems in learning under latent information and nonstationarity in bandits.
- Organizing an interdepartmental social initiative, SPAM (Statistics, Physics, Astronomy, Mathematics).
- Fleshing out ideas for more academic communities like Monsoon Math.
What do I want to learn about/do in the future?
primary goals
- Complete an empirical study of RLHF methods on LLMs of varying size and understand the implementation nuances of major RLHF methods.
- Work on a large scale applied recommender systems project using the latent bandit algorithms that I designed (LOCAL-UCB and ProBALL-UCB).
- Applying ideas from RLHF and bandits to mental health studies that my advisor is involved in.
side-quests
- Design a codenames bot using one LLM and train it againts players designed using a different LLM.
- Explore the nuances of implementing various RL algorithms in simulated motion settings.
- Design meaningful experiments to compare LLM agents trained using language feedback with RL agents trained using numerical feedback, using benchmark frameworks like LLF-bench.
news
Feb 10, 2025 | I have started a Machine Learning Research Internship in the Machine Learning and Inference Research (MLIR) team at Netflix, under Nathan Kallus and Adith Swaminathan! I will be focusing on post-training LLM agents to handle long contexts. I’m excited to see how far we can push the envelope on LLM agents in this internship! |
---|---|
Jan 22, 2025 | Our paper on RLHF under intermediate feedback and partial observability of rewards, A Theoretical Framework for Partially Observed Reward-States in RLHF, has been accepted to ICLR 2025! This is work with Mirco Mutti, Aldo Pacchiano and my advisor, Ambuj Tewari. |
Jun 03, 2024 | I have started my internship at Microsoft Ads, working on ad monetization under my manager Ajith Moparthi and with my mentor Yannis Exarchos! Excited to dive into designing a low latency update algorithm for autobidding models. |
Feb 06, 2024 | Announcing two paper acceptances! My paper on offline reinforcement learning in the presence of confounding, written with Kevin Tan, Yangyi Lu, Maggie Makar, Yixin Wang and my advisor Ambuj Tewari has been accepted to AISTATS 2024. My paper on double descent phenomena in denoising with Rishi Sonthalia and Kashvi Srivastava has been accepted to TMLR 2024. |
Nov 29, 2023 | I have received the Rackham International Student Fellowship, which is offered to 25 students across graduate departments under Rackham! |